Lorenzo Pacchiardi
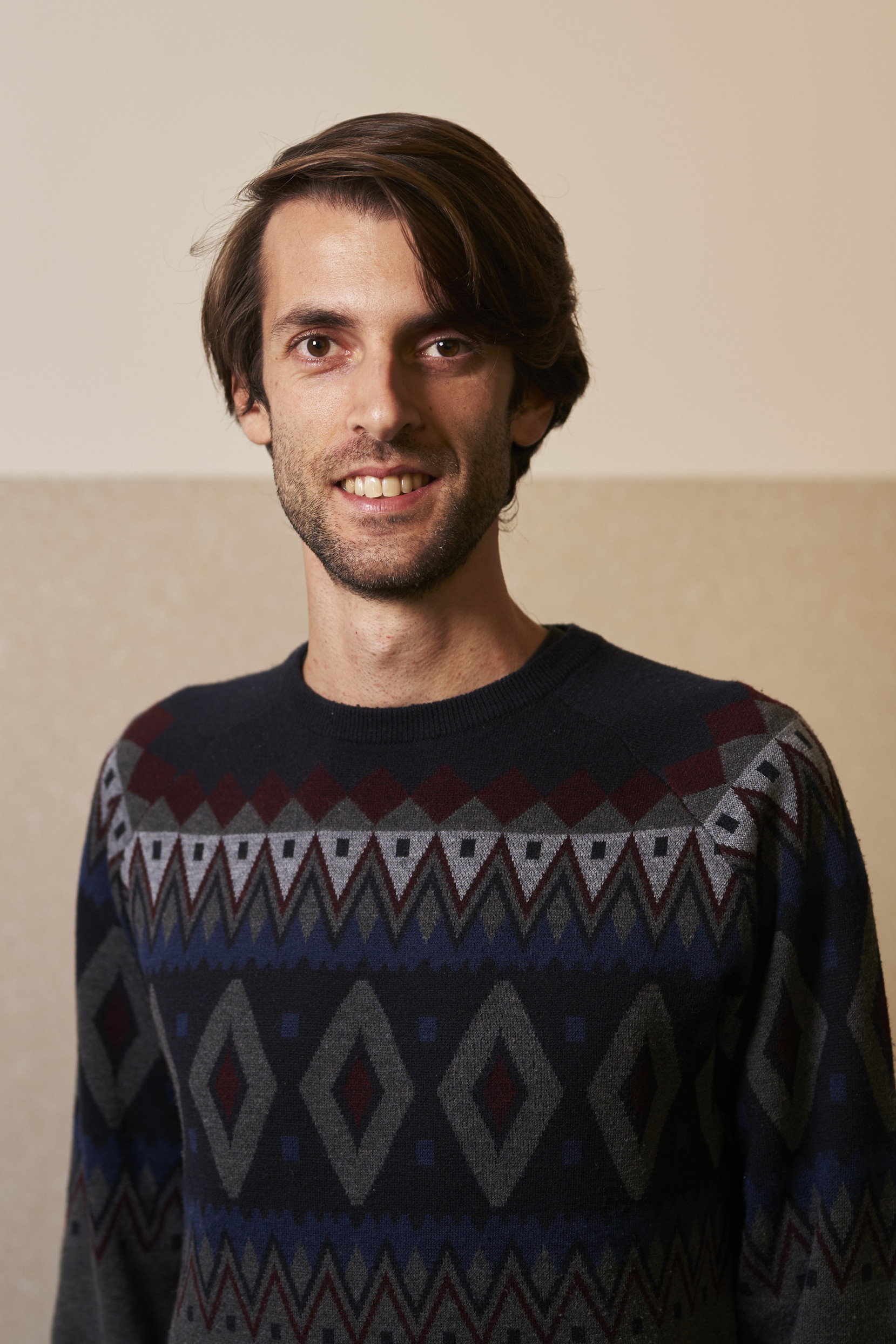
Research Associate, University of Cambridge
I am a Research Associate at the Leverhulme Centre for the Future of Intelligence at the University of Cambridge. I lead a research project (funded by Open Philanthropy) on developing a benchmark for measuring the ability of LLMs to perform data science tasks. I am more broadly interested in AI evaluation, particularly in predictability and cognitive evaluation, and I closely collaborate with Prof José Hernández-Orallo and Dr Lucy Cheke. I contribute to the AI evaluation newsletter.
I previously worked on detecting lying in large language models with Dr Owain Evans and on technical standards for AI for the EU AI Act at the Future of Life Institute. I am deeply interested in AI policy (particularly at the EU level; I participate to the GPAI code of practice drafting process). I also collaborate with The Unjournal to make impactful research more rigorous.
I obtained a PhD in Statistics and Machine Learning at Oxford, during which I worked on Bayesian simulation-based inference, generative models and probabilistic forecasting (with applications to meteorology). My supervisors were Prof. Ritabrata Dutta (Uni. Warwick) and Prof. Geoff Nicholls (Uni. Oxford).
Before my PhD studies, I obtained a Bachelor’s degree in Physical Engineering from Politecnico di Torino (Italy) and an MSc in Physics of Complex Systems from Politecnico di Torino and Université Paris-Sud, France. I carried out my MSc thesis at LightOn, a machine learning startup in Paris.
news
Mar 11, 2025 | Our new preprint shows how to extract the most predictive and explanatory power from AI benchmarks by automatically annotating the demands posed by each question. Check it out! |
---|---|
Feb 21, 2025 | Two new arXiv preprints: one surveying AI evaluation and identifying six main paradigms, the other one introducing a benchmark for jointly evaluating the performance of LLMs and its predictability on individual instances. |
Oct 15, 2024 | We have two new preprints on arXiv! One on predicting the performance of LLMs on individual instances, the other one on predicting the answers of LLM benchmarks from simple features. |
Oct 01, 2024 | I have obtained a grant from Open Philanthropy on building a benchmark for measuring the ability of LLMs to perform data science tasks! 🤓 📊 |
Sep 21, 2024 | Our paper Generalised Bayesian Likelihood-Free Inference (on which I worked during my PhD studies) is now published at the Electronic Journal of Statistics! ![]() |